15 Key Strategies for Effective AI Risk & Compliance Governance
AI Risk & Compliance Governance establishes policies and controls to manage AI-related risks while ensuring compliance with legal, ethical, and regulatory standards. It safeguards data privacy, prevents bias, promotes transparency, and upholds accountability in AI decision-making to mitigate risks and maximize responsible AI value.
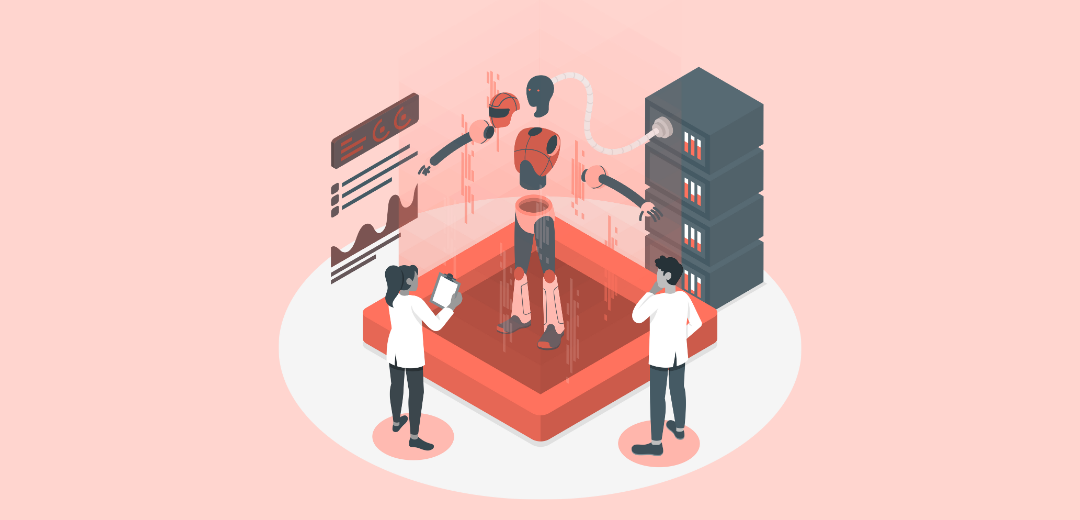
In today’s digital landscape, trust is the most valuable asset for businesses. With the AI market projected to grow twenty fold by 2030, reaching nearly two trillion U.S. dollars, organizations are facing rising expectations from customers, investors, and regulators. The pressure to protect sensitive data, ensure ethical AI use, and maintain security has never been greater. A single lapse—whether it’s a data breach, biased AI decision, or failure to meet compliance standards—can have significant consequences, including reputational damage, financial penalties, and loss of customer trust.
As we move into 2025, digital trust goes beyond cybersecurity. It encompasses transparent data governance, strict compliance, and the responsible deployment of AI systems. A strong digital trust framework is essential for building long-term relationships with stakeholders and adapting to an increasingly complex and evolving digital environment.
A recent report found that organizations’ top concerns around generative AI include data privacy and cybersecurity (65%). Given the growing volume of personal data and the rapid expansion of AI, the need for a robust digital trust program is more urgent than ever. Organizations must be prepared to respond to emerging threats and align with the rising demand for accountability and ethical responsibility.
Read on to learn how organizations can implement a digital trust framework, prioritize data privacy and cybersecurity, and address AI ethics to protect stakeholders and meet regulatory expectations.
What is AI Risk & Compliance Governance?
AI Risk & Compliance Governance refers to the framework of policies, processes, and controls that organizations put in place to manage the risks associated with the use of Artificial Intelligence (AI) while ensuring compliance with legal, ethical, and regulatory standards. This includes safeguarding data privacy, preventing bias in AI systems, ensuring transparency, and maintaining accountability in AI decision-making. Effective governance helps businesses mitigate the potential negative impacts of AI, such as legal penalties, reputational damage, and ethical concerns while maximizing its value in a responsible and compliant manner.
Read: Understanding the Importance and Benefits of Risk Management for Business
Distinguishing AI Risk from Conventional Software Risk
The risks associated with AI and traditional software differ significantly in both nature and impact. While traditional software risks are more predictable and technical, AI introduces unique challenges due to its unpredictability, ethical concerns, and complex decision-making processes.
Aspect | Traditional Software Risk | AI Risk |
Nature of the Risk | Risks are predictable and stem from bugs, errors, security vulnerabilities, and system failures. | Risks are unpredictable, arising from machine learning models, biases, and unknown behaviors. |
Predictability vs. Uncertainty | Issues can be identified and mitigated with thorough testing and debugging. | Uncertainty due to evolving AI models that learn and adapt, leading to unforeseen issues. |
Bias and Ethics | Ethical concerns focus on privacy, data theft, and unauthorized access. | Ethical risks involve AI biases, fairness issues, and lack of transparency in decision-making. |
Complexity and Scalability | Scaling can cause performance issues, but risks are generally well-understood and manageable. | Scalability of AI leads to potential unintended consequences as systems evolve autonomously. |
Accountability and Transparency | Accountability is clear with designated roles for developers and system administrators. | Accountability is murky due to opaque “black-box” models that are hard to interpret and regulate. |
Impact of Failure | Failures tend to be localized, such as system crashes or downtime, with recovery protocols in place. | AI failures can have broader societal impacts, like misdiagnosis, accidents, or perpetuating biases. |
Regulatory and Legal Risks | Software is subject to well-defined regulations, such as data protection and security laws. | New regulations specific to AI are evolving, making compliance more complex and less standardized. |
Let’s explore why a robust AI risk management framework is essential for effectively managing the unique challenges AI introduces. By understanding and managing these risks, businesses can ensure responsible AI implementation and minimize potential negative impacts.
Why You Can’t Overlook an AI Risk Management Framework
According to a 2023 report from McKinsey, 70% of companies globally are using some form of AI, with many planning to increase their investment in AI technologies. As AI continues to become a central part of business operations, the risks associated with its use also grow. A strong AI risk management framework is crucial for businesses to ensure that they can harness the full potential of AI while protecting themselves from potential challenges and disruptions.
1. Compliance with Regulations
As AI technologies evolve, so too do the regulations around them. Governments are increasingly focusing on AI, with laws being enacted to ensure it is used responsibly and ethically. A well-structured risk management plan helps businesses stay compliant with these regulations, preventing potential legal issues or hefty fines.
2. Ethical Considerations
AI has the potential to perpetuate biases, whether in hiring practices, loan approvals, or even customer interactions. Without a framework to manage these risks, businesses may unknowingly create unfair systems that harm vulnerable groups. A solid risk management approach helps identify and mitigate biases in AI models, ensuring that the technology is used ethically and fairly.
3. Safeguarding Data Privacy
AI systems require vast amounts of data to function effectively, and much of this data can be personal or sensitive. Ensuring that this data is protected and handled with care is vital. Risk management in AI helps to safeguard against data breaches or misuse of personal information, helping businesses maintain customer trust and avoid costly penalties.
4. Reducing Operational Risks
AI can be a powerful tool for efficiency, but it’s not without its risks. Without proper oversight, AI systems can make flawed decisions, leading to operational disruptions or financial losses. Risk management frameworks help businesses identify potential failures in their AI systems before they cause serious harm, allowing them to take corrective actions proactively.
5. Strengthening Cybersecurity
As AI becomes a central part of business operations, it also becomes a target for cyberattacks. AI systems are especially vulnerable to adversarial attacks, where malicious actors manipulate AI behavior to their advantage. A comprehensive risk management framework ensures that AI applications are secure from such threats, helping to prevent security breaches and other cyber risks.
6. Protecting Brand Reputation
Lastly, AI-related failures can severely damage a company’s reputation. Whether it’s an algorithm making a biased decision or a data breach exposing customer information, the public’s trust can quickly erode. A strong risk management approach helps companies identify and address issues early on, protecting their reputation and keeping customer trust intact.
In essence, AI risk management is essential for businesses to responsibly harness the power of AI while mitigating its potential downsides. It ensures that AI is used in a compliant, ethical, and secure manner, allowing businesses to thrive in this rapidly changing technological landscape.
Read: What Is the Difference between Risk Control and Risk Management?
How to Identify AI Risks: A Step-by-Step Guide for Businesses
In today’s fast-evolving AI landscape, identifying risks early on is critical to ensure smooth, safe, and effective deployment of AI technologies. Businesses embracing AI must be proactive in managing potential risks that could harm operations, reputations, and customer trust. Let’s break down how you can identify these risks and safeguard your organization from common pitfalls.
1. Understand the Full AI Lifecycle
The first step in identifying AI risks is to take a holistic view of the AI lifecycle—from data acquisition and model development to deployment and ongoing monitoring. At each stage, potential risks may arise. For example, data collection can lead to privacy concerns, model training might introduce biases, and deployment could expose vulnerabilities if not properly tested. By identifying risks at every phase, you can ensure that potential issues are addressed before they become major problems.
2. Use the Right Frameworks for Evaluation
AI risk management requires a structured approach. Frameworks like the NIST AI Risk Management Framework provide comprehensive guidelines for assessing and mitigating risks across various AI systems. These frameworks can help businesses identify potential compliance risks, operational pitfalls, and ethical concerns, ensuring that AI systems are deployed responsibly and transparently. For example, integrating these frameworks into your risk management strategy ensures that AI systems meet regulatory requirements and ethical standards.
Read: Top Practices to Maintain Compliance and Mitigate Regulatory Risks
3. Conduct a Risk Assessment for Each AI Model
Every AI model is different, and so are the risks associated with each. By conducting a thorough risk assessment, businesses can pinpoint specific vulnerabilities for each AI project. This includes evaluating factors like data quality, model transparency, decision-making processes, and the potential for biases. Through continuous testing, businesses can uncover flaws early, before they cause significant harm. This proactive approach ensures that risks are mitigated, compliance is maintained, and AI systems are aligned with ethical standards.
4. Implement Continuous Monitoring for Emerging Risks
AI technologies evolve rapidly, so continuous monitoring is essential. Even after an AI system is deployed, new risks may emerge due to changes in the data it processes or shifts in regulatory landscapes. Regular audits, stress tests, and impact assessments will help keep the system in check, ensuring it stays compliant and effective. Additionally, ongoing monitoring helps identify any vulnerabilities that could lead to cyberattacks or misuse, particularly in sensitive industries like healthcare and finance.
5. Build a Culture of Risk Awareness
Fostering a culture of risk awareness within your organization is just as important as having a formal framework. When all stakeholders—from data scientists to decision-makers—are aware of the risks AI poses, they are more likely to adopt best practices in data handling, model training, and deployment. Creating this culture of accountability ensures that AI systems are not only efficient but also ethical and compliant, reducing risks associated with improper use.
10 Key Areas of AI Risk: What Every Business Should Know
With AI becoming an integral part of modern businesses, identifying and mitigating risks is vital. Below are the 10 key areas of AI risk every business must address to stay compliant, ethical, and secure.
1. Compliance Risk: Staying Ahead of Changing Regulations
AI is a rapidly growing field, and with growth comes regulation. From global standards like the General Data Protection Regulation (GDPR) to industry-specific guidelines, businesses must ensure their AI systems adhere to the latest regulatory requirements. Failing to meet these standards could result in hefty fines or legal consequences. By integrating AI in risk and compliance frameworks, businesses can stay ahead of new regulatory changes and avoid penalties.
2. Data Privacy and Security: Protecting Sensitive Information
AI systems depend on vast datasets, including sensitive personal or organizational information. This makes data privacy and security one of the most pressing risks for AI deployment. Cybersecurity threats such as data breaches or adversarial attacks can expose valuable data and damage trust. Implementing a data governance strategy that includes encryption, anonymization, and strict access controls is essential to protect customer data and ensure compliance with privacy laws.
3. Ethical Risk: Tackling Algorithmic Bias and Discrimination
AI can unintentionally perpetuate biases, leading to unfair or discriminatory decisions, particularly in hiring, lending, or law enforcement. Ethical considerations are critical in AI deployment to ensure fairness and inclusivity. This is why AI ethics and oversight frameworks are necessary. Businesses should regularly audit AI models for fairness and mitigate any biases by diversifying training datasets and refining decision-making algorithms.
4. Transparency Risk: Building Trust through Explainable AI
Lack of transparency in AI decision-making is a significant risk, especially in high-stakes applications. If businesses cannot explain how an AI model made a decision, it may undermine trust, especially in sectors like healthcare or finance. Explainable AI (XAI) aims to make AI decision processes understandable to both end-users and regulators. By adopting explainable models, businesses can enhance accountability and transparency, reducing the risk of legal or reputational damage.
5. Operational Risk: Ensuring Reliable AI Performance
AI models must consistently perform as expected. Operational risks arise when an AI model behaves unpredictably or fails to deliver the desired outcomes. In cases of misaligned models or unforeseen behaviors, operational disruptions could lead to financial losses or safety issues. It’s essential to monitor AI systems in real-time and have contingency plans, including fail-safe mechanisms and human oversight, to prevent such disruptions.
6. Cybersecurity Risk: Guarding Against Malicious Attacks
AI systems are prime targets for cyberattacks. From adversarial machine learning techniques to data poisoning, AI models are vulnerable to exploitation by hackers. AI cybersecurity measures such as adversarial training and regular security audits help mitigate these risks. Businesses should also be prepared for vulnerabilities related to the integration of AI systems into broader IT infrastructures, ensuring comprehensive security at every level.
Read: Understanding Risk Remediation and Management in Cyber Security
7. Model Misalignment Risk: Keeping AI Aligned with Business Goals
AI models can sometimes become misaligned with their original purpose, resulting in decisions that are inconsistent with business objectives or ethical guidelines. For example, an AI optimized for short-term profits may make decisions that harm customers or violate company values. Regular checks and updates are necessary to ensure model alignment with organizational goals. AI governance plays a critical role in ensuring that models remain aligned with the company’s values and mission.
8. Intellectual Property (IP) Risk: Safeguarding AI Innovations
AI technologies are often proprietary and represent valuable intellectual property (IP). Whether it’s a machine learning algorithm, data model, or training data, businesses need to protect their AI innovations from theft or unauthorized use. Companies must ensure that their AI risk management practices incorporate strong IP protection protocols, including securing models, patents, and contracts with third-party vendors.
9. Liability Risk: Determining Responsibility in AI Failures
As AI makes more decisions on behalf of businesses, the question of accountability becomes increasingly important. Who is liable if an AI system makes a mistake that leads to financial loss, harm to individuals, or legal violations? Developing a clear liability framework ensures that businesses are prepared to handle such scenarios, assigning responsibility where it’s due and protecting themselves from legal consequences.
10. Social and Environmental Impact: Mitigating AI’s Broader Implications
AI’s effects extend beyond business operations and could have profound social and environmental consequences. From potential job displacement to environmental damage caused by the energy consumption of AI models, businesses must consider AI’s social impact and work towards solutions that foster a positive societal change. Incorporating AI into sustainable development efforts and addressing social inequalities ensures responsible use of AI technologies.
The potential of AI is undeniable, but with great power comes significant responsibility. Addressing these 10 areas of AI risk management not only ensures compliance and security but also builds a foundation of trust and ethical integrity. By taking a proactive approach to identifying and mitigating AI risks, businesses can unlock the full potential of AI while avoiding costly mistakes and safeguarding their reputation.
Check out the Top 10 Risk Management Softwares for 2025
15 Key Strategies for Effective AI Risk & Compliance Governance: Avoiding Common AI Risk Management Failures
1. Establish a Risk-Based Classification System for AI Systems
AI systems, like any technology, present varying levels of risk depending on their application. High-risk systems—such as those used in healthcare, finance, or legal settings—demand more robust oversight, governance, and compliance frameworks than low-risk systems like those used for marketing automation.
Why It Matters:
Without a risk-based classification system, businesses may either over-regulate low-risk AI, stifling innovation, or fail to apply sufficient scrutiny to high-risk applications, leading to potential legal and ethical breaches.
What You Should Do:
Begin by categorizing AI models based on their potential societal or operational impact. Use the EU AI Act as a reference for risk-based classification, which outlines different risk categories and regulatory requirements. Apply stricter governance measures to high-risk AI systems, such as comprehensive auditing, regular bias checks, and human oversight protocols.
2. Adopt Continuous AI Risk Monitoring and Audit Tools
AI systems are dynamic; they continuously learn from new data, which means their behavior can change over time. Continuous monitoring ensures that risks such as model drift, data privacy violations, and security breaches are detected early, minimizing the chances of compliance failures.
Why It Matters:
AI models in production might evolve unexpectedly, which means risks such as bias, errors, and non-compliance could emerge after deployment. If not caught early, these risks can escalate into major issues for your organization, including legal penalties and reputational damage.
What You Should Do:
Implement AI risk monitoring platforms that track compliance metrics and model performance in real-time. These tools should monitor for deviations in model behavior, ensuring that AI systems continue to align with compliance and governance standards. Use automated auditing tools to maintain transparent records of AI decisions and actions, especially in high-risk areas like healthcare or finance.
3. Implement Explainable AI (XAI) for Transparency
Explainable AI is essential for ensuring that AI systems remain accountable, especially in sectors where decisions have significant implications, such as healthcare and criminal justice. By making AI decisions more understandable, businesses can prevent issues related to “black-box” algorithms.
Why It Matters:
Transparency in AI decision-making is crucial to avoid trust erosion among stakeholders and to ensure compliance with emerging AI regulations, such as the EU AI Act and GDPR. Lack of transparency could lead to regulatory penalties and harm your business reputation.
What You Should Do:
Adopt Explainable AI (XAI) methods to make the inner workings of AI models clear to users, auditors, and regulators. Use model interpretability techniques that allow you to explain how decisions are made, particularly when AI systems are used in high-stakes applications. This is critical not only for regulatory compliance but also for internal accountability.
Read: Risk Management in Business: Best Practices and Trends for 2025
4. Enforce Data Privacy and Protection Standards
As AI systems rely heavily on vast amounts of data—often personal or sensitive—data privacy is one of the most critical aspects of AI compliance. Properly managing and protecting data from unauthorized access or breaches is essential to avoid regulatory fines and to maintain consumer trust.
Why It Matters:
With data protection laws like GDPR and CCPA, companies are legally required to ensure the privacy and security of personal data used in AI systems. A data breach or misuse of personal data can result in substantial fines, legal ramifications, and a loss of public trust.
What You Should Do:
Implement strict data privacy and security protocols, including data encryption, anonymization, and secure access controls. Ensure that only necessary data is collected for AI training by adopting data minimization principles. Perform regular data audits to ensure that AI systems are compliant with data protection regulations. Leverage privacy-enhancing technologies (PETs) to secure sensitive information processed by AI models.
5. Institutionalize AI Ethics Oversight and Bias Mitigation
AI has the potential to perpetuate societal biases present in training data, which can result in discriminatory decisions. Implementing a robust ethics and bias mitigation strategy is critical to ensuring fairness and avoiding legal challenges.
Why It Matters:
Unchecked biases in AI can lead to discrimination in areas like hiring, lending, and law enforcement. Ethical risks, including bias and inequality, can damage public trust, violate regulations, and expose your business to litigation.
What You Should Do:
Develop an ethics committee or board to oversee the ethical use of AI. Implement bias detection and mitigation techniques, such as fairness audits, during model training and throughout the AI lifecycle. Ensure diversity in the data used to train models to avoid biased decision-making. Use established frameworks like the OECD AI Principles to guide AI ethics policies and ensure compliance with fairness and accountability standards.
6. Adopt a Structured AI Governance Framework
AI governance is crucial to ensure that AI systems align with business objectives, regulatory requirements, and ethical standards. A structured framework provides clarity on the roles, responsibilities, and processes for AI management across the organization.
Why It Matters:
Without a clear governance structure, organizations risk inconsistent AI implementation, which can lead to compliance failures, security lapses, and operational inefficiencies.
What You Should Do:
Establish an AI governance committee or team to define policies, set accountability measures, and manage risks. Leverage frameworks like the NIST Risk Management Framework to establish guidelines for implementing and monitoring AI systems. This should include clear roles and responsibilities for data scientists, IT professionals, compliance officers, and leadership teams.
7. Use AI for Real-Time Compliance Monitoring
AI technologies can be harnessed to monitor and ensure compliance with regulations continuously. By automating compliance checks, businesses can ensure that AI models remain aligned with evolving regulatory frameworks and ethical guidelines.
Why It Matters:
AI’s rapid pace of development requires a continuous assessment to ensure that systems remain compliant as they evolve. Automated tools can detect non-compliance faster than manual efforts, reducing risk and providing real-time alerts to potential issues.
What You Should Do:
Use AI-powered compliance monitoring tools that integrate with existing AI models. These tools can track regulatory changes and assess AI system behavior, flagging any discrepancies in real-time. This proactive approach ensures that your AI systems remain compliant, transparent, and secure throughout their lifecycle.
8. Ensure Accountability with Human Oversight
AI decisions should never be fully autonomous, particularly in high-risk areas. Human oversight is necessary to validate AI decisions, ensure that they meet ethical standards, and comply with legal requirements.
Why It Matters:
Lack of accountability in AI-driven decisions can lead to costly mistakes, regulatory violations, and ethical dilemmas. Human oversight ensures that AI remains a tool for enhancing decision-making rather than replacing human judgment in critical areas.
What You Should Do:
Implement a “human-in-the-loop” process for critical decisions. This means that AI-generated recommendations must be reviewed and validated by qualified personnel before implementation. Ensure that the individuals overseeing these processes are trained in AI governance and compliance to ensure the highest standards of accountability.
9. Foster a Culture of AI Risk Awareness
AI governance and risk management are not the sole responsibility of compliance teams—they must be ingrained in the company’s culture. A company-wide commitment to responsible AI use is essential for minimizing risks and ensuring compliance.
Why It Matters:
Without widespread awareness, AI risks may be overlooked, leading to non-compliance, biased decisions, or ethical violations. A responsible AI culture promotes informed decision-making and active participation across all departments.
What You Should Do:
Conduct regular training for employees at all levels to ensure they understand AI risks, ethical considerations, and compliance requirements. Encourage collaboration between AI developers, business units, and compliance teams to foster an environment of shared responsibility for AI governance.
10. Create an AI Risk Management Roadmap
To manage AI risks effectively, businesses need a clear and comprehensive roadmap that outlines goals, processes, and timelines. This roadmap should be flexible, allowing adjustments as AI technologies, regulations, and risks evolve.
Why It Matters:
Without a clear risk management roadmap, businesses may struggle to prioritize AI risk mitigation efforts, leading to reactive rather than proactive strategies. A roadmap provides a structured approach to anticipate and address emerging risks.
What You Should Do:
Develop an AI risk management plan that includes clear milestones for identifying, assessing, and mitigating AI risks. This should align with your organization’s broader strategic objectives and be regularly updated to reflect new technologies and regulatory changes.
11. Implement Comprehensive AI Compliance Audits
Regular audits are vital to ensure that AI systems comply with legal, regulatory, and ethical standards. These audits help identify vulnerabilities, biases, and gaps in governance that could lead to non-compliance.
Why It Matters:
AI audits help uncover hidden risks that could lead to significant financial and reputational damage. Consistent auditing also ensures that AI systems remain aligned with organizational values and regulatory frameworks throughout their lifecycle.
What You Should Do:
Schedule periodic audits of AI systems to assess compliance with data privacy laws, ethical guidelines, and regulatory requirements. Audits should cover data sources, decision-making logic, and model performance to ensure full compliance.
Read: The importance of risk assessment and risk management
12. Engage with Regulators to Stay Ahead of AI Legislation
As AI regulations evolve, staying engaged with regulatory bodies is crucial to ensuring compliance and avoiding penalties. By participating in consultations and contributing to the development of new AI laws, businesses can better prepare for upcoming requirements.
Why It Matters:
Regulatory landscapes are shifting rapidly, and proactive engagement helps businesses anticipate and adapt to changes, avoiding fines and maintaining compliance.
What You Should Do:
Regularly engage with industry associations and regulatory bodies to stay informed about emerging AI laws and participate in public consultations. Use these insights to adjust your AI governance strategies and ensure that your AI practices align with current and future regulations.
13. Mitigate AI Cybersecurity Risks
As AI becomes an integral part of business operations, it also becomes a prime target for cyberattacks. Adversarial attacks and model manipulation are growing threats.
Why It Matters:
AI systems are vulnerable to manipulation, which can have disastrous consequences, especially in industries like finance, healthcare, and defense.
What You Should Do:
Implement cybersecurity measures specifically designed for AI, including adversarial attack detection and model integrity checks. Regularly test AI models for vulnerabilities and ensure that all data processing complies with the highest security standards.
Read: Cybersecurity Risk Avoidance: Proactive Strategies to Safeguard Your Organization
14. Develop a Change Management Strategy for AI Compliance
AI systems, regulations, and business needs evolve over time, so having a change management strategy for AI compliance is critical. This strategy ensures that as AI technologies and regulatory requirements change, your business can adapt smoothly.
Why It Matters:
Without a change management process, businesses may fail to adapt to new regulations, causing non-compliance and missed opportunities.
What You Should Do:
Implement a change management protocol that includes regular reviews of AI governance policies, updated training, and flexible compliance systems. This ensures that AI systems stay compliant as regulations evolve.
Read: Understanding What is a Risk-Based Approach
15. Foster Innovation with Regulatory Sandboxes
While regulatory compliance is critical, overly stringent regulations can hinder innovation. Regulatory sandboxes allow businesses to test AI technologies in controlled environments to understand their impact and compliance requirements.
Why It Matters:
Sandboxes provide a safe space for testing innovative AI solutions while ensuring that they comply with regulatory standards.
What You Should Do:
Explore the use of regulatory sandboxes to pilot AI technologies. Work with regulators to understand the specific conditions under which your AI innovations can be tested, ensuring compliance without stifling creativity.
By adopting these strategies, businesses can mitigate AI-related risks and ensure compliance across their AI operations. A comprehensive AI governance strategy ensures transparency, accountability, and ethical decision-making, positioning companies to thrive in the rapidly evolving AI landscape.
Managing AI Risks with VComply
Organizations need a connected, modern platform to handle the evolving risks of AI. VComply offers a comprehensive solution for managing AI-related risks while ensuring compliance with legal and ethical standards.
- Centralized Risk Management
VComply’s Risk Register allows organizations to record and track all AI risks in one place, ensuring nothing slips through the cracks. By categorizing risks and assigning owners, businesses gain full visibility over their AI risk landscape. - Collaborative Risk Workshops
Leverage Risk Workshops to bring key stakeholders together and collaborate on mitigating AI risks. This ensures that risks are assessed from all angles, and effective solutions are developed collectively. - Automated Risk Assessments
With automated workflows, VComply simplifies the process of managing AI risk assessments, ensuring consistent evaluations and timely identification of new risks. This proactive approach reduces the risk of non-compliance and strengthens oversight. - AI-Specific Risk Templates
VComply’s pre-built risk templates tailored for AI applications help businesses stay aligned with global AI compliance standards. These templates streamline the assessment of AI systems for ethical issues, security, and privacy. - Strategic Dashboards and Reporting
VComply’s interactive dashboards offer real-time insights into AI risks, allowing you to visualize trends and make informed, strategic decisions quickly. - Alerts and Escalation
Receive timely alerts for high-priority AI risks, ensuring quick action and reducing the chance of unmanaged impacts.
By integrating VComply, organizations can enhance AI risk management and ensure their AI systems meet evolving regulatory and ethical standards, all within a single platform.
The 7 Best GRC Systems Redefining Compliance and Risk Management in 2025
Wrapping Up
AI governance and risk management are no longer optional for organizations looking to thrive in an AI-driven world. With the rapid advancement of AI technologies, businesses must take proactive measures to mitigate risks, ensure compliance, and maintain ethical standards. From implementing clear governance frameworks and leveraging automated tools to fostering a culture of accountability, each step you take toward better managing AI risks will not only protect your organization but also set it up for long-term success.
At the core of this process is the ability to continuously monitor, assess, and adapt your AI systems to align with regulatory changes and ethical requirements. The strategies outlined here—coupled with the power of a centralized platform like VComply—enable organizations to stay ahead of AI risks and navigate the complex regulatory landscape efficiently. To get started on your journey towards more effective AI risk management, sign up for a 21-day free trial of VComply today and discover how our platform can help streamline your compliance and risk management efforts.